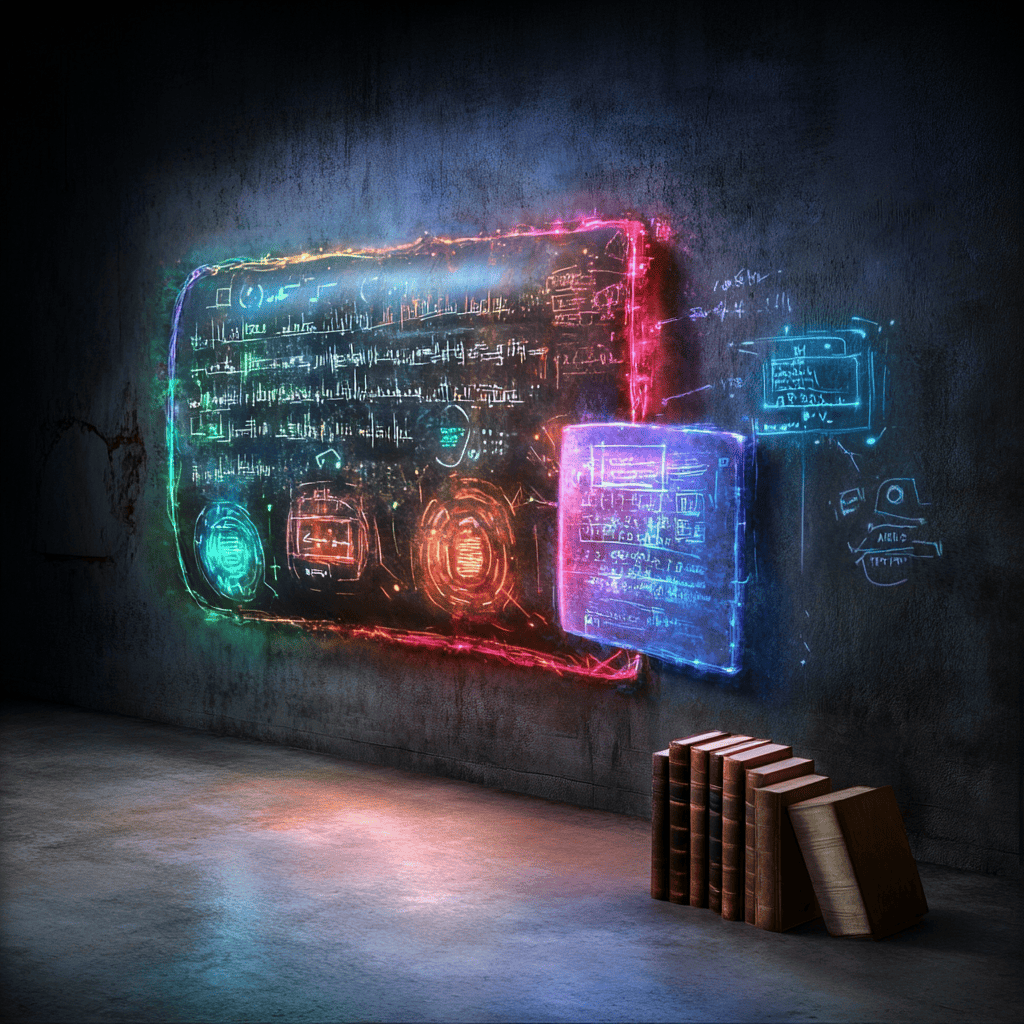
For years, we’ve heard that AI will revolutionize education—but is it really living up to the promise? A new editorial, Don’t Believe the Hype: AI Myths and the Need for a Critical Approach in Higher Education, takes a deeper, more skeptical look at AI’s role in learning, warning that blind enthusiasm could be just as dangerous as outright rejection.
So, where does AI help, where does it fall short, and what should educators and institutions be focusing on? Let’s break it down.
The AI Revolution in Higher Education: Reality vs. Myth
🛑 Myth #1: AI is a self-sufficient, all-knowing entity.
✅ Reality: AI depends on human ingenuity, labor, and data extraction—often at an exploitative scale. It’s not an independent force of nature; it’s a tool shaped by human biases and priorities.
🛑 Myth #2: AI will democratize education and level the playing field.
✅ Reality: While AI can expand access to education, it often magnifies existing inequalities. Disparities in data quality, algorithmic biases, and access to AI-powered tools can actually reinforce social and economic divides.
🛑 Myth #3: The US has an insurmountable lead in AI.
✅ Reality: China is catching up—fast. While some argue AI won’t have a major impact on jobs, automation and Generative AI (GAI) are deepening economic divides globally.
🛑 Myth #4: AI-driven learning is inherently superior.
✅ Reality: AI may be great at automating tasks, but it struggles with nuance, creativity, and ethical judgment—all of which are essential for critical thinking and higher education.
The Hidden Risks of AI in Universities
While AI has the potential to enhance education, the report warns that its widespread adoption comes with serious risks, including:
⚠️ Academic Integrity Challenges – AI-generated essays and automated grading can erode evidence-based teaching and make plagiarism detection harder.
⚠️ Over-reliance on AI Tools – Universities risk outsourcing thinking to AI, producing graduates who lack essential critical analysis skills.
⚠️ Data & Privacy Concerns – Large-scale data extraction raises ethical concerns about who owns student-generated data and how it’s used.
⚠️ Bias & Misinformation – AI isn’t neutral; it can amplify biases, reinforce stereotypes, and misinterpret context—potentially leading to misinformation in educational settings.
A Smarter Approach: Critical AI Literacy in Education
🔹 AI shouldn’t replace education—it should enhance it. The focus must be on teaching students how AI works, its limitations, and when to trust it.
🔹 AI literacy should be embedded into curricula. Universities need to ensure that students and faculty understand the ethical, technical, and societal implications of AI.
🔹 A balanced approach is key. Rather than blindly adopting every AI tool, educators should critically evaluate AI’s impact on learning, fairness, and student outcomes.
🔹 Invest in human-AI collaboration. The goal isn’t to replace educators but to augment human intelligence—allowing professors to focus on mentoring, creativity, and personalized learning.
The Bottom Line: AI Is Not a Magic Fix for Education
AI isn’t a one-size-fits-all solution for higher education. While it can streamline tasks and enhance learning experiences, it also poses significant risks that need careful oversight and ethical consideration.
The real challenge isn’t just implementing AI—it’s making sure it actually serves students, educators, and society as a whole. How do we ensure AI is used responsibly in education?
📖 Source: Don’t Believe the Hype: AI Myths and the Need for a Critical Approach in Higher Education